I would guess that many readers of this blog have heard of the 2020 paper “Are Ideas Getting Harder To Find?” by Nicholas Bloom, Charles Jones, John Van Reenen, and Michael Webb. This paper is at the center of current debates around stagnation, singularity, and progress. Bloom et al document a serious problem with economic growth: our investments into research are growing fast but the rate of economic growth is constant at best. This observation is hard to explain within the prevailing theory and it suggests that maintaining current rates of economic growth will become impossible if our population of researchers ages and shrinks. The authors posit that the declining return on research investment is due to something inherent about exploring ideas that gets more costly the more ideas you already know.
I think that their data on declining returns to research investment are correct and important, but their assumptions about what explains this decline are as unsupported by the data as the assumptions of the original theory they are trying to amend. The best way to understand this paper from a layman’s point of view is through a metaphor.
An Extended Metaphor
Imagine we’re studying a car. Our best understanding of how this car works comes from Paul Romer, the greatest mechanic in the land. His model goes something like this: Fuel goes into the engine where it’s combusted and transformed into a force spinning the driveshaft of the car. The force from the driveshaft then goes through the tires which interact with the road surface, pushing the car forwards. Our best model assumes that a constant level of fuel use should lead the car to have a constant acceleration rate. For example, if the engine gets a gallon of gas every hour, the car’s speed will increase by 1% every hour.
A relationship like this would lead to unbounded exponential growth in the car’s speed. If fuel use was somehow positively related to the car’s speed (maybe there’s a forced induction turbocharger), there would be a positive feedback loop, and the car’s speed would grow even faster than exponential over time. This doesn’t sound like any car we’re familiar with but hey, if you look how the speed of particular vehicle has changed over time, it looks like a pretty good model.
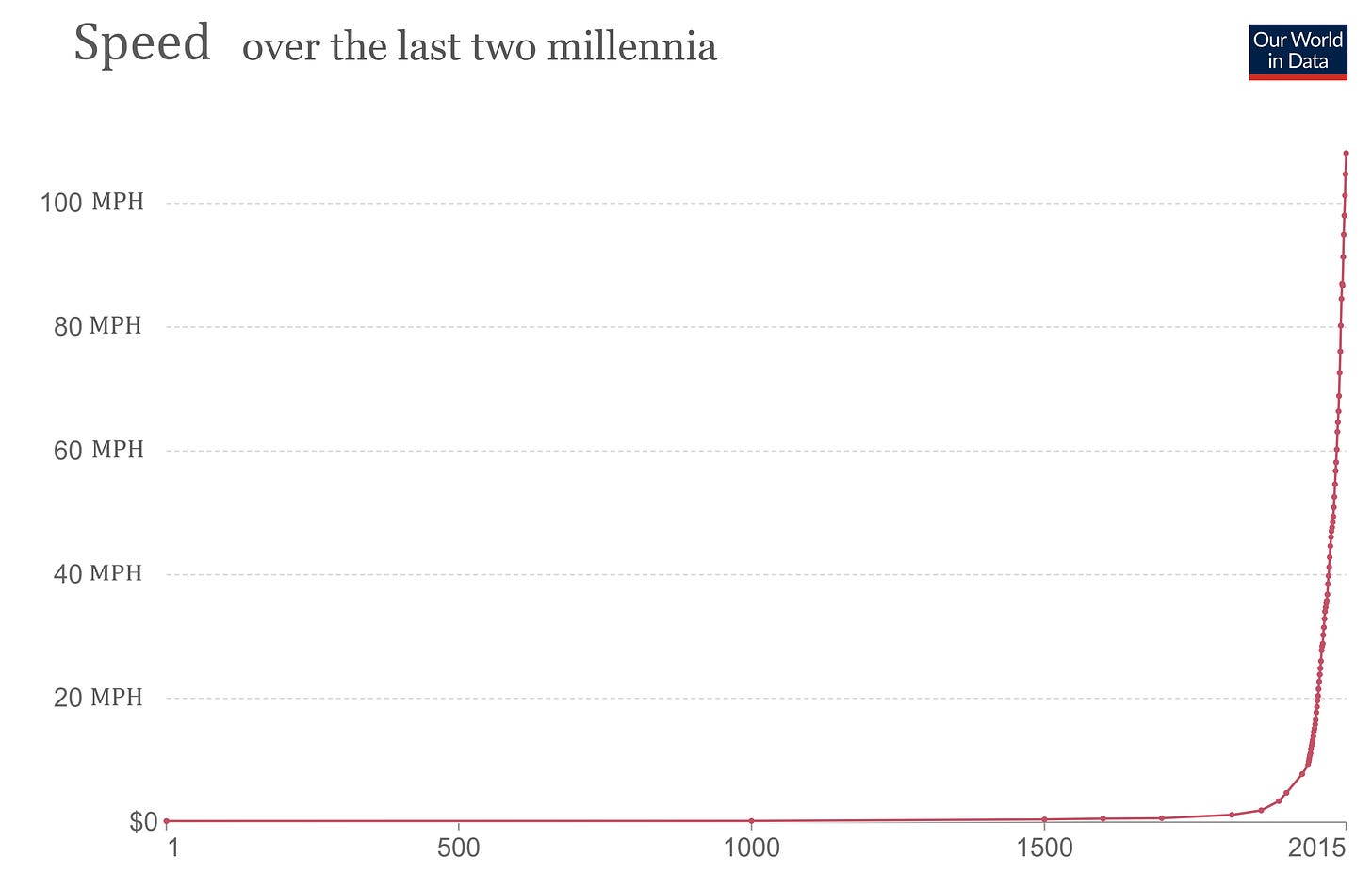
Now, the young mechanics Bloom, Jones, Reenen, and Webb come along looking skeptical. “Sure” they say, in a chorus of voices, “Romer’s model of constant fuel use ==> constant acceleration rate looks good when you zoom out to this low resolution across all of history, but lets look at the past 100 years where we have better data.”
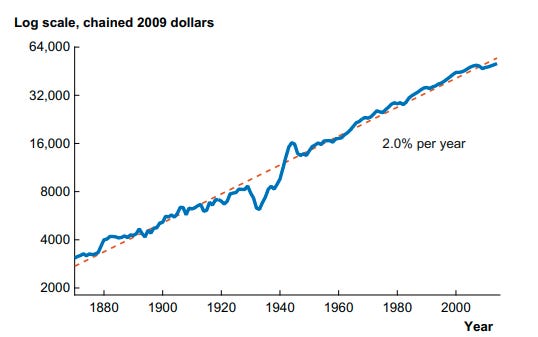
Bloom, Jones, Reenen, and Webb each hold up this graph and say, in unison, “Acceleration has been constant over this entire period, it’s no longer speeding up as it seems to have been in the past.”
Paul Romer, the greatest mechanic in the land, retorts “But this is perfectly consistent with my theory! We observe constant acceleration and this is exactly what I assume will arise from constant fuel use.”
“Ah ha!” Bloom et al cry in four-part harmony, “this is just what we expected you to say. Indeed your theory predicts constant acceleration from constant fuel use, but take a look at this:”
“While acceleration has been constant over the whole period, fuel use is growing fast!” Paul Romer, the greatest mechanic in the land, is stunned into silence as Bloom, Jones, Reenen, and Webb continue their graphical hymn. “Today, each gallon of gas accelerates the car 30x less than it used to. Far from each unit of fuel having a constant effect on acceleration, fuel has been becoming less productive for 100 years.”
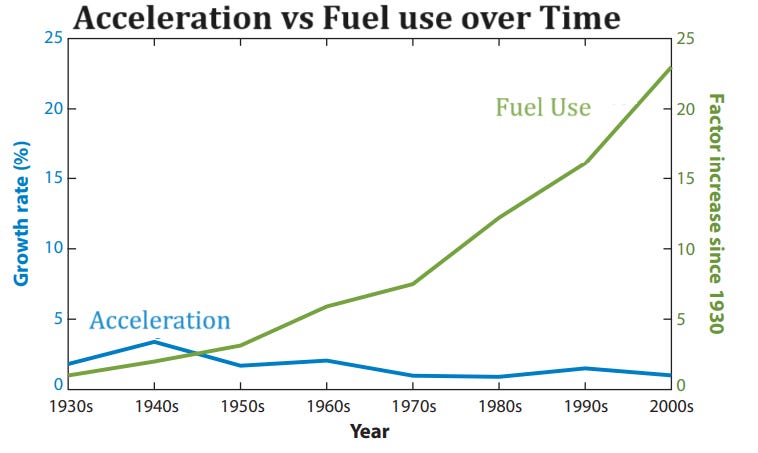
Bloom et al let their voices echo over the silent crowd of mechanics who have gathered round, studying the charts. The silence dripped from the stage for so long it built up stalactites between the onlookers. Finally the four voices began again, quietly now, “Therefore, we propose a new model. Accelerating the drive shaft by a fixed percentage does not take a fixed amount of energy. The fuel input required to accelerate by 1% increases as the speed of the drive shaft increases. It takes more fuel to double the speed of a flywheel if its already spinning fast. As speed increases, acceleration gets harder to find.” At this, Paul Romer instantly crumbles into dust. Anticipating the next question the four new greatest mechanics in the land quickly continued. “By how much does the fuel input required to accelerate the drive shaft increase? Well, we need only look at our data. The old paradigm was that acceleration, the derivative of speed, was directly proportional to the fuel use. Like this:” Bloom, Jones, Reenen, and Webb each pull out a chalk board and start scribbling.
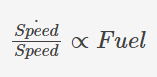
“But now we know that this cannot be the case! The right side of this equation has increased by 20x but the left side has stayed the same, so they can’t be directly proportional. Since we have observed increasing fuel use and constant change in speed, it must be that constant fuel use leads to decreasing change in speed. Thus:”
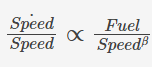
“The old paradigm held that β = 0, but to explain the divergence between fuel use and acceleration β must be around 3! Each percent increase in the growth rate of speed gets harder to find very rapidly as speed increases.”
Back To Reality
This extended metaphor should give you a good sense of the context and purpose of the “Are Ideas Getting Harder to Find?” paper in academic economics. Replace speed with economic growth and fuel with R&D spending and the discussion is the same.
Paul Romer’s original model of economic growth assumed that a constant population of researchers would produce a constant growth rate in the economy. For example, if our economy has a million researchers working every year, it’s output should grow by 1% every year. Bloom et al noticed that the number of researchers in our economy has grown to 23 times its size in 1930 but the growth rate of the economy has been constant, even decreasing, over that period. So Paul Romer’s assumption that constant research input ==> constant growth rate seems unsupported by the data. Bloom et al explain the divergence between growing research input and constant economic growth by assuming that ideas get harder to find as we discover more of them.
But both sides of this economic modeling debate are missing something important. When you’re driving a car, there is usually a close relationship between the gas pedal and your acceleration. More pedal, more fuel, more acceleration. If you observe a divergence between your fuel use and your acceleration, what should you think? One explanation might be that there are just inherent diminishing marginal returns in the system, that’s what Bloom et al use to explain the divergence. But you might just be going up a hill. Or you turned on to a rougher road. Or your engine is depreciating and it needs repairs. Or some combination of all of these. Without looking around at the road conditions or pulling over to pop the hood, it’s premature to pick on explanation over the other.
This is the situation we’re in with science. We see a divergence between research inputs and productivity outputs. One explanation might be that there are just inherent diminishing returns to searching idea-space. Maybe the burden of knowledge makes each expansion of the frontier harder because scientists need to cover the ground of all who came before them if they want to extend knowledge further. Or maybe we have picked all of the low-hanging fruit and we only have hard ideas left. These are intuitive reasons why ideas might get harder to find as we discover more of them, but before we conclude anything about the inherent properties of idea production we need to pull over and see if there are other explanations.
What does that look like in the context of science? Well, take a look at the model behind the “Are Ideas Getting Harder to Find?” paper.
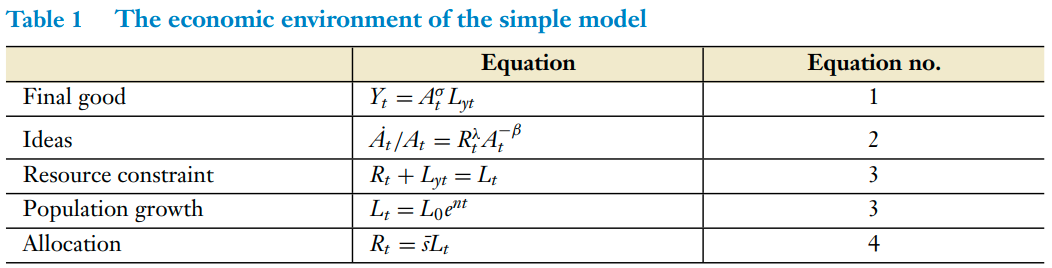
The first two equations are the most important. Equation 2 is the first step in the car model. The “fuel” (researchers R) is turned into acceleration of the “drive shaft” (technology A). Including the A^-β term in equation 2 is what differentiates this model from Paul Romer’s original where β = 0. When β>0 “ideas get harder to find” because as our stock of ideas (A) gets larger, our fuel (R) gets divided by a larger and larger number (A^β). Notice that R is also raised to a power, delta. This represents the returns to scale of researchers in idea production. Doubling the number of researchers might more than double the output of economic growth because they all specialize and trade and have knowledge spillovers which make them more productive than the sum of their parts. Or, doubling researchers might less than double economic growth because researchers compete for the same grants and repeat work that others have already done.
Equation 1 is the “Final Good” production function. In our car metaphor this is where the rubber hits the road, literally (metaphorically). There are returns to scale here too. Doubling the force on the driveshaft might more than double the speed of the car on downhill asphalt, but not on a steep climb up a dirt road. Similarly, doubling the stock of ideas (A) might more than double economic output if ideas can be implemented freely and synergize with each other. But if technology adoption is resisted by culture or regulation then you won’t see much growth even if good ideas are abundant.
Bloom, Jones, Reenen, and Webb show evidence that research inputs (R) are growing rapidly while observed technological progress (A^σ) grows at a slow constant rate. But this pattern can be reproduced by an infinite number of combinations of the three parameters we just talked about. The authors assume that the returns to scale on researchers and ideas are constant, then dub β the “ideas are getting harder to find” parameter and attribute all the divergence to this factor alone.
The divergence between growing research inputs and stagnant economic growth is definitely worth pointing out and studying. Something in this production function is getting harder. Diagnosing why we aren’t getting as much growth a we think we should is important for the well-being of billions of human lives. But the evidence presented in “Are ideas getting harder to find?” is not enough to answer in the affirmative. In future posts we’ll look at evidence which can help us decide between different explanations for this slow down, but the intention of this post is to highlight that the question is still open and to inspire curiosity about what the reasons might be.
I am pretty convinced that β>0, even though I also think there is some contribution to slowdown from other factors. Some reasons:
That said, I am also pretty convinced that there is a contribution from institutions and other factors. (To paraphrase something Eli Dourado said to me, TFP growth is slow in Venezuela, and no one thinks that is because ideas are harder to find in Venezuela.)
I basically agree with everything you've said here.
On the subdomains point, you can have decreasing returns within each subdomain but constant returns overall if you keep finding new subdomains. I think this is an accurate model of progress. It captures ideas like paradigm shifts and also integrates the intuitions for low-hanging fruit and burden of knowledge in a way which still allows rapid progress. My favorite example is the Copernican revolution. There were huge obstacles from burden of knowledge and low-hanging fruit in Ptolemaic astronomy. It took so much extra data and education to improve the epicycles of Mercury by a few decimal points. But once astronomy moved to a new model, there was a whole new grove of low hanging fruit and almost none of the investment in Ptolemaic astronomy was necessary to make progress so the burden of knowledge was reset.
Absolutely true that new subdomains open up new areas of low-hanging fruit. It is the “stacked S-curve” model.
Not immediately clear whether what this means for β>0. I think this model may be addressed in Bloom et al, or maybe in an earlier paper by Jones. I vaguely recall that it doesn't make a difference whether you analyze things in terms of the subdomains or the economy at large, but I don't have the exact reference at hand.
I agree with your conclusion, Maxwell, and this piece was a joy to read. Jason's comment also seems correct to me in that subdomains very clearly exhibit the phenomenon of ideas getting harder locally. Still, the fallacy of composition tells us to be wary of summing up these subdomains. Diversification across subdomains may the answer to how the innovation frontier can continue to expand despite ideas getting locally more challenging.
I'm curious to hear what you think is the scarce resource. After trying my hand at starting a company and working in venture capital, I've come to appreciate that often the idea is quite important. The old hobbyhorse of execution vs idea feels like a false dichotomy though. The best companies do not spring forth from entrepreneurs' heads like Athena, fully dressed for battle, but they are also not A/B tested into existence. Similarly, science seems to move forward through a combination of dogged empirical work and theoretical insight.
Here are a few areas I'd like to read more about: courage, ignorance/fools and subversion/tricksters. I always think of the strange case of Medicine Nobel Dr. Barry Marshall, who debunked long established medical beliefs about stress being the cause of gastric ulcers by performing risky self experimentation that involved infecting his gut with bacteria: https://asm.org/Podcasts/MTM/Episodes/The-Self-Experimentation-of-Barry-Marshall-MTM-144
Maybe I'm missing something, but this post doesn't seem to actually back up the (strong!) claim in its title. Seems more like you're defending "Something Is Getting Harder To Find But It May Or May Not Be Ideas".
No you're right, I had a section in there evaluating the different possible reasons for slow down but it got too long so that will be coming in future posts. Sorry for the hyperbolic title!