Thoughts on Dario Amodei’s “Machines of Loving Grace.”
By Niko McCarty for Asimov Press.
Dario Amodei, the CEO of Anthropic, recently published an essay called “Machines of Loving Grace.” It sketches out his vision for how AI could radically transform neuroscience, economics, diplomacy, and the meaning of work. Amodei also imagines the ways AI could accelerate biological research and yield miraculous cures in the 21st century; everything from the prevention and treatment of nearly all infectious and inherited diseases to the elimination of most cancers.
“Biology is probably the area where scientific progress has the greatest potential to directly and unambiguously improve the quality of human life,” Amodei writes. “My basic prediction is that AI-enabled biology and medicine will allow us to compress the progress that human biologists would have achieved over the next 50-100 years into 5-10 years.”
This is an inspiring vision, but as Amodei acknowledges, achieving it will first require that we think deeply about existing bottlenecks and then roadmap ways to solve them. Indeed, most of what we publish at Asimov Press are roadmaps of this kind, including essays that examine persistent obstacles to scientific progress (such as “Where’s the Synthetic Blood?”) or speculative fiction that imagines possible futures once these obstacles have been overcome (see “Tinker” or “Models of Life”).
Amodei’s essay considers what might happen to biological research should a “powerful AI” emerge that is “smarter than a Nobel Prize winner.” However, it isn’t clear that such a superintelligence could even be applied to its full potential in biology today, given the dearth of high-quality datasets needed to train it.
Although Amodei does acknowledge some real-world issues limiting scientific progress — such as the slow growth of organisms and tedious clinical trials — he mostly passes over the more general tools that will be required to accelerate research in the near term. Still, many of the bottlenecks slowing biology today are biophysical, rather than computational. Therefore, I’m using Amodei’s essay as a rallying cry for researchers to innovate their way past existing bottlenecks in wet-lab biology, which, if achieved, would help scientists actually build more powerful AI models in the future.
It wasn’t easy for me to write this essay because it’s often difficult to predict exactly where a solution to a given problem will emerge. That’s why researchers hoping to accelerate biology at large should strive to build “platform tools” that “can apply in multiple contexts,” as Adam Marblestone has written, rather than narrow solutions to short-term problems.
The balance between AI advances on the one hand and wet-lab innovations on the other is also a bit like the chicken and egg problem. Yes, AI will accelerate biological progress, but first we must make it easier and faster to run experiments while creating better methods to study biology in a more holistic and less reductionist way. Solving the latter challenges will, oddly enough, require both machine learning and wet-lab innovations.
To understand what I mean by “biophysical” bottlenecks, just consider the ongoing quest to build a virtual cell. This is one of the biggest ambitions amongst biologists today, and more than a hundred scientists — at Arc Institute and the Chan Zuckerberg Initiative, for example — are working to build models that can accurately simulate living organisms. A virtual cell would enable researchers to perform biology research on computers, rather than actual organisms, thus removing challenges that arise from working in the “world of atoms.” But the creation of a virtual cell is not possible by computation alone; we’ll first need to understand much more about how cells work, using real-world experiments.
Today, we are largely unable to predict the outcomes of even “simple” biological questions, such as, “If I take this gene from an Arabidopsis plant, and insert it into Escherichia coli, will the cell express the gene and make functional protein?” Nobody really knows without trying it out in the laboratory.
In order for 50-100 years of biological progress to be condensed into 5-10 years of work, we’ll need to get much better at running experiments quickly and also collecting higher-quality datasets. This essay focuses on how we might do both, specifically for the cell. Though my focus in this essay is narrow — I don’t discuss bottlenecks in clinical trials, human disease, or animal testing — I hope others will take on these challenges in similar essays.
Bottlenecks
In his recent essay, Amodei cites several bottlenecks that hinder our ability to make progress in biological research. “The main challenges with directly applying intelligence to biology,” he writes, “are data, the speed of the physical world, and intrinsic complexity (in fact, all three are related to each other). Human constraints also play a role at a later stage, when clinical trials are involved.”
In this essay, I’m going to focus on just two of these: the speed of experiments and the complexity of biological systems. I’ve chosen these two because I think they are the linchpin by which we’ll be able to build broadly useful AI models for cell and molecular biology. Such models will, in turn, make it much easier to invent the sorts of platform tools that Amodei asserts “drive >50% of progress in biology,” such as CRISPR and mRNA vaccines, because the creation of these tools ultimately derive from a deeper understanding of how cells work.
By solving these bottlenecks, we’ll also be able to collect the data required to build a virtual cell, thus enabling scientists to conduct experiments in silico rather than in the world of atoms. This would further accelerate the speed and scale at which we can run experiments, enabling “more shots on target” and quicker testing of hypotheses. Scientists are already building a model that can, for example, look at which RNA molecules are expressed in a cell at t=0 and predict how those molecules will change at t=1. But these models will become increasingly sophisticated over time.
Even more fundamentally, a virtual cell is a way to encapsulate the current extent of our biological knowledge. By measuring what a real cell does and then comparing those measurements to predictions made by a virtual cell, we can more easily rally resources and direct talent to resolve shortcomings in knowledge. One of my ultimate dreams in life is to see, before I die, a virtual cell that can accurately simulate the real thing.
An apt comparison for this pursuit of a virtual cell is the hydrogen atom in physics, which has become something of a universal language for that field. The hydrogen atom enabled physicists to build a quantum theory of matter. And because lots of physicists were working with the same atom, they could easily compare results between experiments to construct foundational theories.
Biology has no such common language. The cell is the most basic unit of life, and yet it evades our understanding. Even E. coli, the most widely-studied microbe of all time, has hundreds of genes with unknown functions, and we cannot predict how mutations in, say, genes A+B+C will affect the phenotype of the organism. By “solving the cell” then, I think biologists will find their hydrogen atom and, over time, construct a foundation of knowledge upon which successive generations can build. Again, this can be done by speeding up experiments and making better measurement tools.
It’s worth noting that Amodei, as best I can tell, agrees that these two issues are the major “physical,” or wet-lab, bottlenecks, slowing biology today. Near the top of his essay, he writes:
Experiments on cells, animals, and even chemical processes are limited by the speed of the physical world: many biological protocols involve culturing bacteria or other cells, or simply waiting for chemical reactions to occur, and this can sometimes take days or even weeks, with no obvious way to speed it up … Somewhat related to this, data is often lacking — not so much in quantity, but quality: there is always a dearth of clear, unambiguous data1 that isolates a biological effect of interest from the other 10,000 confounding things that are going on, or that intervenes causally in a given process, or that directly measures some effect (as opposed to inferring its consequences in some indirect or noisy way). Even massive, quantitative molecular data, like the proteomics data that I collected while working on mass spectrometry techniques, is noisy and misses a lot (which types of cells were these proteins in? Which part of the cell? At what phase in the cell cycle?).
So let’s consider the first bottleneck: Why are experiments in biology so slow? There are a few reasons. For one, life itself grows and develops slowly, and the many steps required to “do” biological research in the laboratory are often tedious and manual.
For example, cloning DNA molecules and inserting them into cells — a process required for basically all experiments in molecular biology — takes up to a week of work. This is because cloning involves multiple steps, including amplification of the DNA molecules, pasting them into loops of DNA, and then inserting the manipulated molecules into living cells. Each of these steps takes several hours to a day.
Biology experiments are also bottlenecked by the growth of organisms. Diseases like Alzheimer's manifest over several decades, making it challenging to study their progression within single individuals in any manageable timeframe. Aging unfolds over an entire lifespan, complicating efforts to understand and intervene in age-related decline. Even in vitro experiments — or those done in the laboratory — are relatively slow because E. coli divides about every 20 minutes under optimal conditions. Human cells divide much more slowly; about once every day.
Most biology experiments are also really expensive, which limits the number of people who can work on a given research problem. This means that biologists often run fewer experiments than they ideally would and thus have fewer chances to answer research hypotheses. Synthesizing a single human protein-coding gene costs several hundred dollars and even a simple PCR machine (used for amplifying DNA) costs between $1,500 and $50,000.2 Many scientists have found innovative ways to lower these costs, but doing so also takes time away from other experiments they could be working on.
The speed of experiments is likely the greatest bottleneck to biology research as a whole because this fundamentally limits the number of ideas we can test, as Sam Rodriques, CEO of FutureHouse, has written. But looking ahead to the second bottleneck, what does it mean to say that biology is “complex”?
Molecular biology has only been around for about 80 years, and tools to study biomolecules (such as DNA and RNA sequencing, or proteomics) have only existed for about half of that time. We are in the absolute infancy of molecular and cell biology, and this means there’s a lot of stuff we still don’t understand. Even the humble E. colihas about 4,400 protein-coding genes,3 in addition to hundreds of types of other biomolecules, including lipids, carbohydrates, and metabolites. Each molecule presumably has some important function in the cell, but current technologies only allow us to measure a tiny fraction of them at any given time. Biologists seeking to build a cohesive knowledge base are basically stuck trying to piece together reductionist observations.
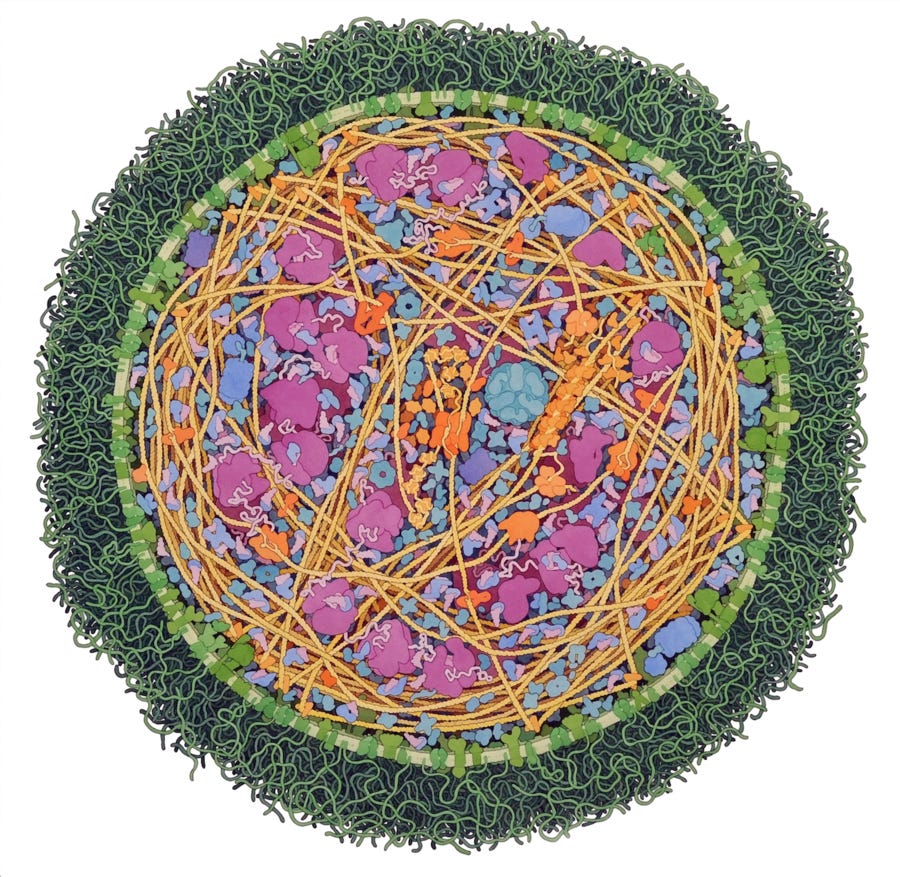
Similarly, we don’t really know how cells change dynamically across space and time. Most methods to study a cell’s biomolecules merely produce static snapshots, failing to capture the short-lived, temporal fluctuations underlying everything from gene expression to molecular signaling pathways. This lack of dynamism limits our ability to build broadly predictive computational models. Fortunately, people are working on solving this problem.
If we can overcome these two bottlenecks, biological research will accelerate. Researchers will be able to collect more holistic and dynamic datasets, as well as run more experiments much faster, to eventually build virtual cells that accurately simulate cellular functions in silico. Such a trajectory isn’t necessarily AI-limited — though presumably AI could make it faster or more efficient.
Faster Experiments
A minor improvement to a widespread method can radically change the world. One way to speed up the pace of discoveries, then, is to think about the fundamentals, including the cost of raw materials and the existing rate limits of ubiquitous methods.
Even a minor acceleration (say, 10 percent) in the speed of PCR, gene cloning, or CRISPR gene-editing, for example, could speed up the pace of biological research as a whole. There are more than 500,000 life sciences researchers in the U.S., so widespread adoption of a faster tool could collectively save millions of hours of research effort per year.
One way to speed up experiments is literally to make biology go faster, or to remove the “slow stuff” from experiments entirely. DNA cloning and many other methods require, for example, that scientists first “grow up” DNA molecules by replicating them inside of living cells, such as E. coli. If we could get E. coli to grow twice as fast — or find ways to clone genes without using organisms at all — then we could shave off a day or two from experiment times. Just because E. coli “evolved” to divide every 20 minutes does not necessarily mean that these cells are biophysically unable to grow faster.
Cutting the size of the E. coli genome could reduce replication times because a smaller genome can be copied more quickly. Cells could also be “artificially evolved” in the laboratory by selecting for mutations that favor faster growth rates. Or, scientists could even drop E. coli entirely and begin using fast-dividing organisms plucked from nature. Vibrio natriegens, for example, was discovered in a salt marsh on Sapelo Island, off the coast of Georgia state, and doubles every 9.4 minutes.
Another way to speed up experiments is to reduce costs, making it so that more scientists are able to work on a problem or so that experiments can be automated entirely.
The cost of “raw materials” in biology is currently far too high. Perhaps you’ve seen this chart before, which shows the falling costs of DNA sequencing over the last two decades. More specifically, it reveals that the price to sequence a human genome fell from $100 million in 2001 to $700 by 2021; a stunning collapse in price.
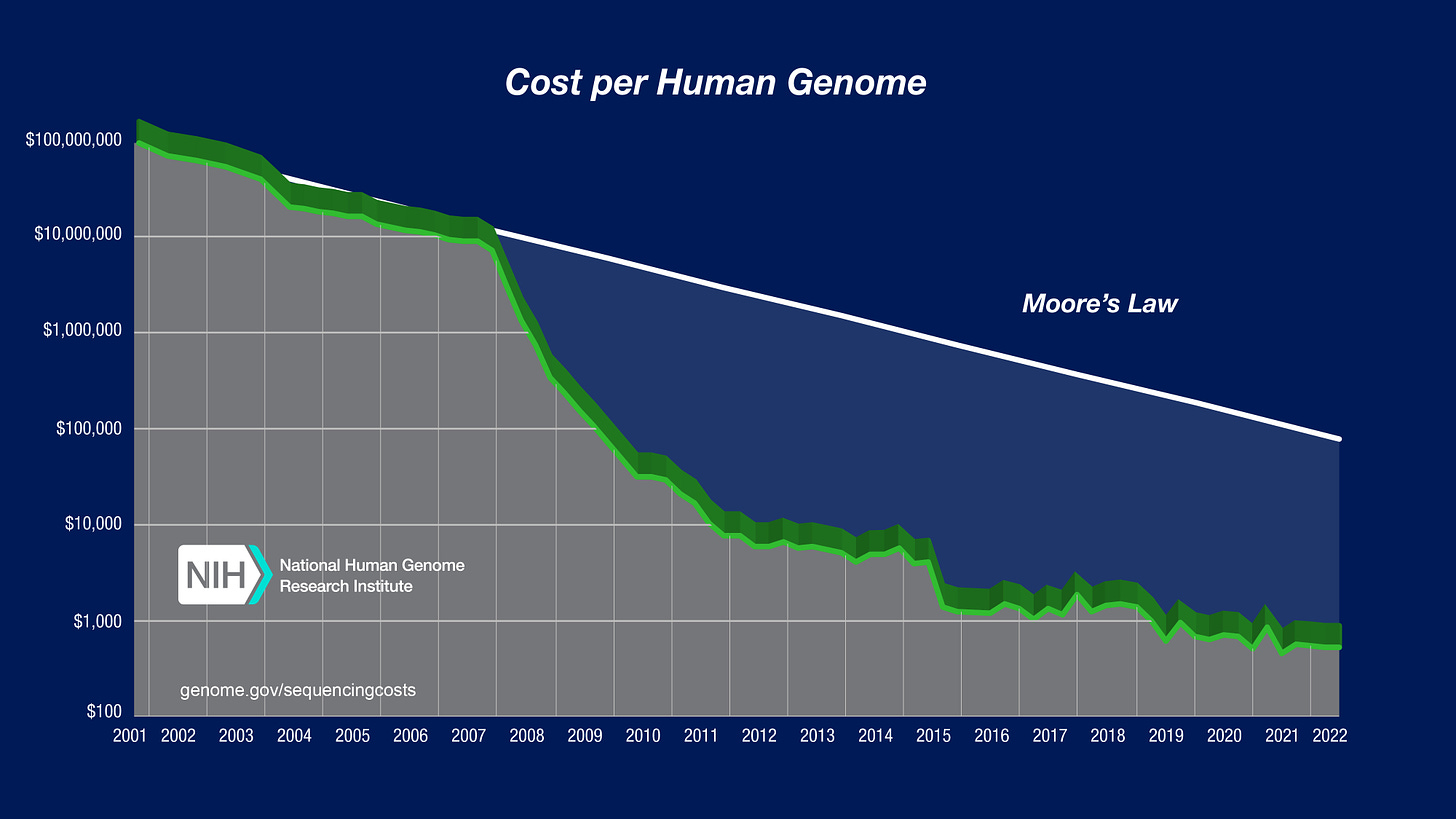
But fewer people seem to think about the next chart, which shows how costs of DNA synthesis have fallen over a similar period. In 2000, it cost about $20 to make one base of DNA; an A, T, C or G. Today, the same feat can be done for about $0.10. A 200x drop in price over twenty-five years is impressive, but these costs have now flatlined — or have even risen — since 2020. Decreasing the cost of DNA by another 100x could be one of the best ways to accelerate experiments because DNA is a fundamental ingredient in so many of them.
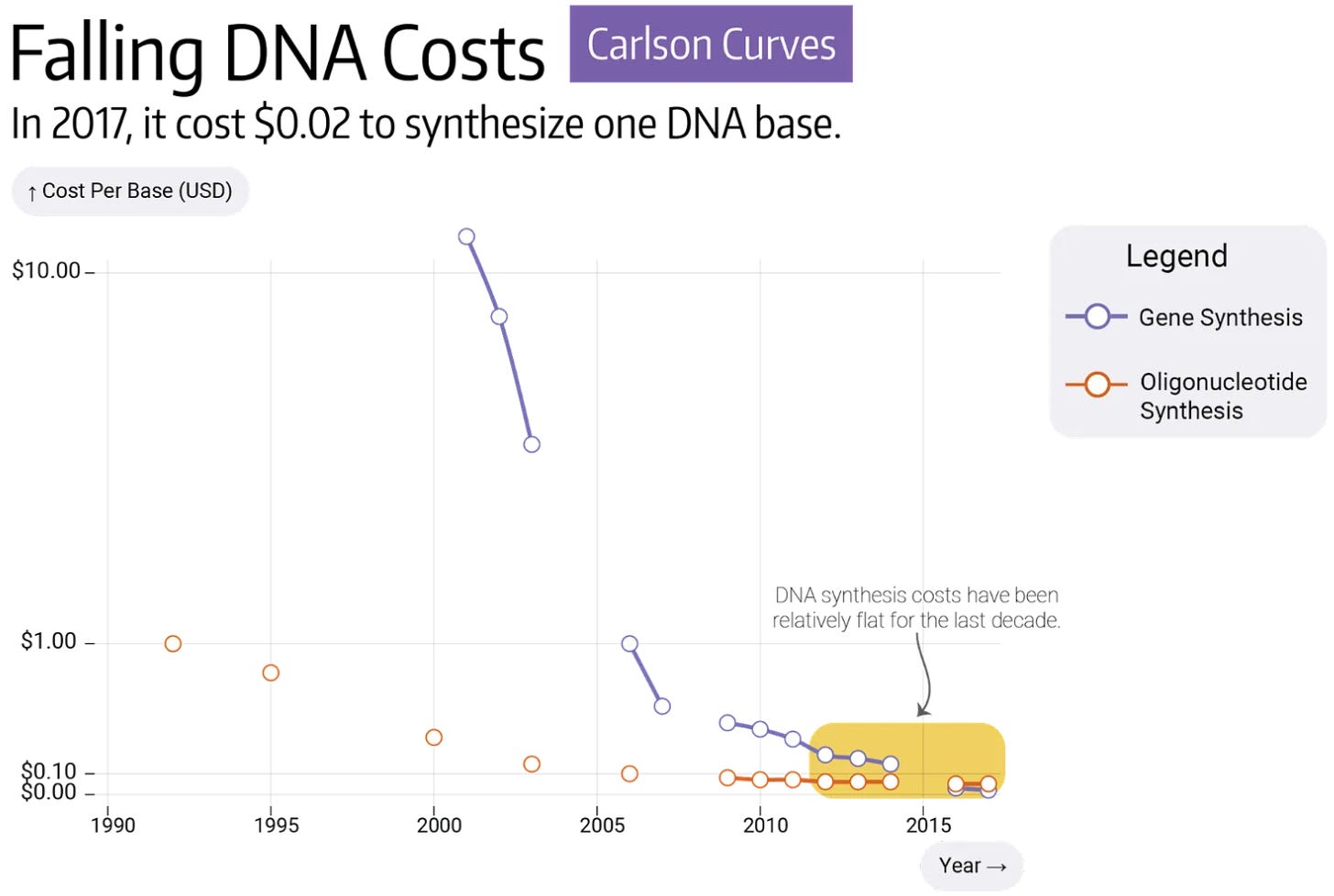
Even protein design, an exciting area of research in which scientists use computers to design proteins that don’t exist anywhere in nature, relies heavily upon DNA synthesis. David Baker, who just shared part of this year’s Nobel Prize in Chemistry, has designed proteins that bind to pathogens or to the membranes of cancer cells. More recently, his team designed proteins capable of “growing” semiconductors by nucleating zinc oxide. In other words, they made an initial step toward biologically-designed GPUs.
Making a computer-designed protein in the laboratory requires, first, that scientists synthesize a string of DNA encoding the protein, insert that DNA into living cells, and then isolate the proteins churned out by the engineered cells. If a protein has 1,000 amino acids, then Baker’s team must first build a DNA molecule stretching 3,000 nucleotides in length (three nucleotides are called a “codon,” and each codon encodes one amino acid in the final protein.) Buying such a molecule from a DNA synthesis company costs hundreds of dollars. But if DNA synthesis becomes cheaper — and emerging technologies suggest that it could — scientists will be able to design, make, and test more proteins in the laboratory.4
In cases like this, it may be possible to find creative ways to circumvent the cost of raw materials entirely. One could theoretically make a device that creates custom proteins without relying upon DNA synthesis at all — an idea I’ve proposed previously.5 Or, we could “industrialize and automate protein purification and testing in a more centralized way,” says Adam Marblestone, or build more accurate computational models such that scientists don’t need to test so many proteins in the first place.
Regardless, as the costs to run experiments fall, we’ll be able to automate more of them, testing hypotheses and collecting larger datasets. But I’m not convinced this will happen in the short term because the outcomes of even common experiments are often unreplicable. Biologists must frequently repeat and troubleshoot experiments, and doing so wastes time and resources. It’s not clear that this troubleshooting can be done with AIs in the near-term, either, as Sam Rodriques has written:
A note on lab automation: chemistry may be all heat, weighing, and mixing, but keep in mind that we recently discovered after 6 months of investigation that one of the projects in my lab [at the Crick Institute in London] was failing because a chemical that was leaching out of a rubber gasket in one of our cell culture wells was interfering with an enzymatic reaction. Full parameterization means the robot needs to be able to pick up the Petri dish and notice that there is some oily residue floating on the surface that isn’t supposed to be there. Until then, humans will probably stay significantly in the loop.
Complex Biology
If, as Amodei may imagine, AI-based models could one day be used to reliably simulate the entirety of a living cell or rapidly speed up the pace at which biologists design tools to precisely control cells, then we’ll first need to collect much better data about how cells actually function.
Cells are complex, in part, because they vary greatly over space and time. Atomic bonds are measured in Angstroms (10-10 meters), molecules in nanometers, cells in micrometers, and animals in meters — biological systems span more than ten orders of magnitude. Experiments performed at one scale don’t always produce data that are informative for experiments at other scales. Cells are also densely packed and the biophysical interactions within them are highly stochastic and probabilistic. (Biophysicists often write their equations using statistical mechanics, calculating probabilities to model the behaviors of large collections of molecules.)
Biology operates over wide time scales, too, and this limits the tools we can use to answer fundamental questions. An experiment designed to study chemical reactions, for example, doesn’t necessarily apply to questions about evolution. Chemical reactions occur at the nanosecond scale, gene expression changes over the second timescale, and evolution occurs over days (for microbes) or years (for animals).
Historically, biologists have studied one type of biomolecule at a time — such as DNA or proteins — and often only at a single moment in time. And now, in the 21st century, we’re left with this tangled mess of reductionist observations that, somehow, we must assemble into a cohesive whole.
To understand what I mean, consider DNA. As I previously wrote for The Latecomer:
In 1990, the U.S. Department of Energy and National Institutes of Health initiated a 15-year plan to map ‘the sequence of all 3.2 billion letters’ of DNA within the human genome. A first draft was published in 2003; it was a patchwork of sequenced DNA from multiple people. The sequence helped scientists identify thousands of disease-causing mutations and understand the evolution of Homo sapiens by comparing DNA with that of Neanderthals’. But still, a genome sequence is simply a string of letters—what those letters actually mean is a far more difficult question.
It’s now clear that not just the sequence of bases, but also the physical structure, contributes to the meaning of DNA. In the last twenty years, data captured using a method called Hi-C has revealed large chromosome chunks that preferentially touch other chromosome pieces in the genome.6 Certain cancers and developmental disorders arise through errors in how these genes physically fold up in a cell.
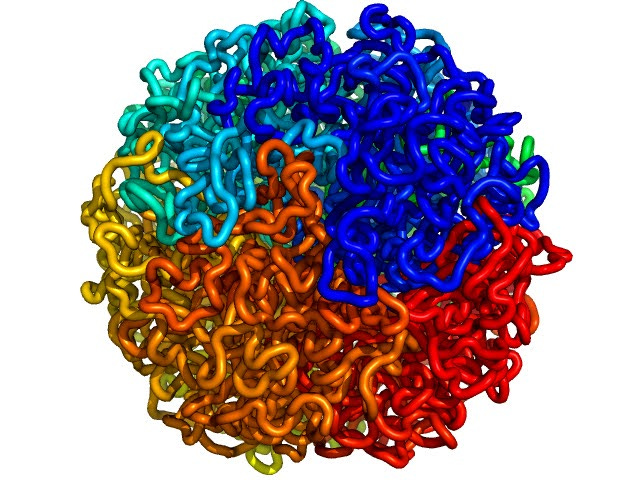
In other words, life does not operate according to a one-dimensional language etched in As, Ts, Cs, and Gs — the letters of the genome — nor is DNA the only material that dictates cellular behavior. Though the genome might be compared to the order of notes in a piece of music, it is missing the dynamics and tempo.
So even though it is relatively simple today to insert large chunks of DNA into a genome, construct customized strands of DNA, and sequence millions of different organisms — feats that would have been unimaginable to most biologists just two decades ago — the messy reality is that we often don’t know what we’re looking for in all that sequencing data, nor can we predict which genes should be edited to make a cell do what we want it to do. In short, our ability to read DNA has outstripped our ability to understand DNA.
Although one gene may encode one protein in the cell, for example, that protein can then play roles in dozens of other cellular processes. The genome is “at best an overlapping and potentially scrambled list of ingredients that is used differently by different cells at different times,” as Antony Jose, a cell biologist at the University of Maryland, has written.
A cell’s proteins often play roles in many different pathways because that is what evolution favors; signaling networks are made to be robust, but not always reliable, so that they can adapt to sudden changes. If one deletes a protein-coding gene from a cell, the cell will often be “okay” because other proteins “fill in” to quell the damage. Proteins belonging to the Akt family, for example, have distinct yet overlapping functions, playing roles in protein synthesis, cellular division, and protein recycling. When scientists deleted the gene encoding a major Akt protein in mice, called Akt1, about 40 percent of the animals died as neonates while the other 60 percent survived and appeared entirely normal. It’s surprising that some of these animals are able to withstand the loss of such an important gene.
Because proteins are so promiscuous, predicting how a signaling network actually works — and thus how most multicellular organisms actually behave — is also nearly impossible from genetic information alone, as bioengineer Devon Stork has written.
In other words, to understand the connections between genotype and phenotype, even in a single cell, we must first build better tools to measure fluxes — how cells change over time — and other dynamic processes. We’ll also need to invent better ways to build DNA, insert it into cells, and test how those cells behave, as Jason Kelly, the CEO of Ginkgo Bioworks, has said.
Fortunately, many biologists agree that our measurement tools have been lacking and have been working earnestly to improve them. Scientists have developed techniques to freeze and image entire cells, for example, using a technique called cryo-electron tomography, or cryo-ET.
Cryo-ET can image cellular structures in three dimensions under near-native conditions. A cell is frozen in place, and electrons are used to capture images from multiple angles. Scientists then use computer algorithms to combine these images and reconstruct detailed 3D models with resolutions up to 4 nanometers.
One problem with this method, though, is that even a single cell is too thick for electrons to penetrate. But scientists today are using ion beams to shave off layers of the cell and thin it down to a diameter less than 300 nanometers, so electrons can pass all the way through. This technique "opens large windows into the cell’s interior," according to a 2013 review, allowing visualization of hidden structures that can’t be easily extracted or crystallized.
For example, cryo-ET has already been used to solve the structure of the nuclear pore complex, a giant cluster of interlocking proteins that weigh 125 million Daltons and are made from about 30 different nucleoporin proteins that control which molecules can pass in and out of the cell’s nucleus. Using cryo-ET and ion beams, researchers visualized the nuclear pore complex in its native nuclear envelope, revealing that it has eightfold rotational symmetry that would have been impossible to observe with any other method. Researchers at the Chan Zuckerberg Institute for Advanced Biological Imaging are also steadily releasing tools to make cryo-ET better.
Another (simpler) way to study cells is with optical expansion microscopy, which now has a resolution of 1 nanometer. The gist is that polymer gels are inserted into a biological specimen — like a slice of brain — that swell and physically expand the sample. Rather than increase the resolution of microscopy, in other words, expansion microscopy enables researchers to increase the size of the actual specimen.
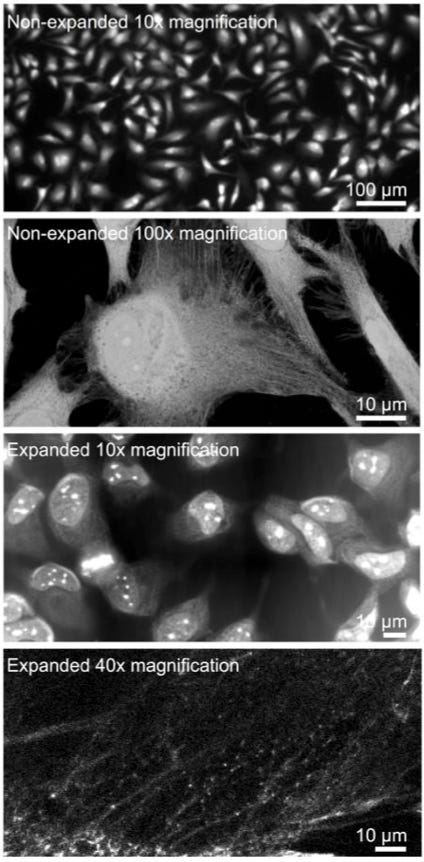
Similar advancements are occurring in techniques to study individual biomolecules across space and time, too.
SeqFISH, developed by Long Cai’s group at Caltech, maps the spatial positions of thousands of RNA, DNA, or protein molecules within single cells. It uses fluorescent probes that hybridize to specific sequences, and through sequential rounds of hybridization and imaging, assigns each target molecule a unique barcode. The method can detect up to 10,000 genes simultaneously with sub-diffraction-limit resolution.
More recently, Michael Elowitz's group — also at Caltech — published a method to study changes in RNA molecules within living cells. They engineered genetically-encoded RNA exporters, based on viruses, that package and secrete RNA molecules in protective nanoparticles, allowing non-destructive monitoring of those RNA molecules in real-time. It’s similar, in many ways, to a technique called Live-seq, wherein scientists extract small amounts of cytoplasm — as little as 1 picoliter — from cells using a micropipette and then sequence the captured RNA molecules at specific time points. Live-seq similarly enables scientists to study RNA expression, without killing cells, over time.
Overall, the last decade or so has been a golden age in biological measurements. The challenge now will be in pushing these methods further still and then reconciling all the datasets to build a comprehensive understanding of how cells work — or at least feeding them into models that can do that job for us, without understanding per se.
Gene Dreams
More people should care about “biological progress” and work towards the visions described by Amodei. This century absolutely can be the best century in the history of humanity, if we make it so.
“Death rates from cancer have been dropping ~2% per year for the last few decades,” writes Amodei. “Thus we are on track to eliminate most cancer in the 21st century at the current pace of human science.” But that’s not all:
Given the enormous advances against infectious disease in the 20th century, it is not radical to imagine that we could more or less ‘finish the job’ in a compressed 21st … Greatly improved embryo screening will likely make it possible to prevent most genetic disease, and some safer, more reliable descendant of CRISPR may cure most genetic disease in existing people … life expectancy increased almost 2xin the 20th century (from ~40 years to ~75), so it’s ‘on trend’ that the ‘compressed 21st’ would double it again to 150. Obviously, the interventions involved in slowing the actual aging process will be different from those that were needed in the last century to prevent (mostly childhood) premature deaths from disease, but the magnitude of change is not unprecedented.
In other words, the future of biology has the potential to be far greater than its past; and the past was pretty good!
Smallpox was declared eradicated by the World Health Organization in 1980 when, just thirty years prior, it afflicted about 500,000 people annually. Similarly, just forty years ago, about 8,000 pounds of pancreas glands harvested from 24,000 pigs were required to make just one pound of insulin, an amount sufficient to treat 750 diabetics for one year. The world’s supply of insulin is now made by engineered microbes. And one hundred years ago, most people with hemophilia died by 13 years of age because, at the time, there was no way to store blood; the only treatment option was to transfuse blood from a family member. Today, a single injection of an FDA-approved gene therapy, called Hemgenix, cures this disease.
In the last 60 years, crop yields have also more than doubled such that we can now theoretically feed 10 billion people with our current food supply, without chopping down any more forests or clearing any more land.
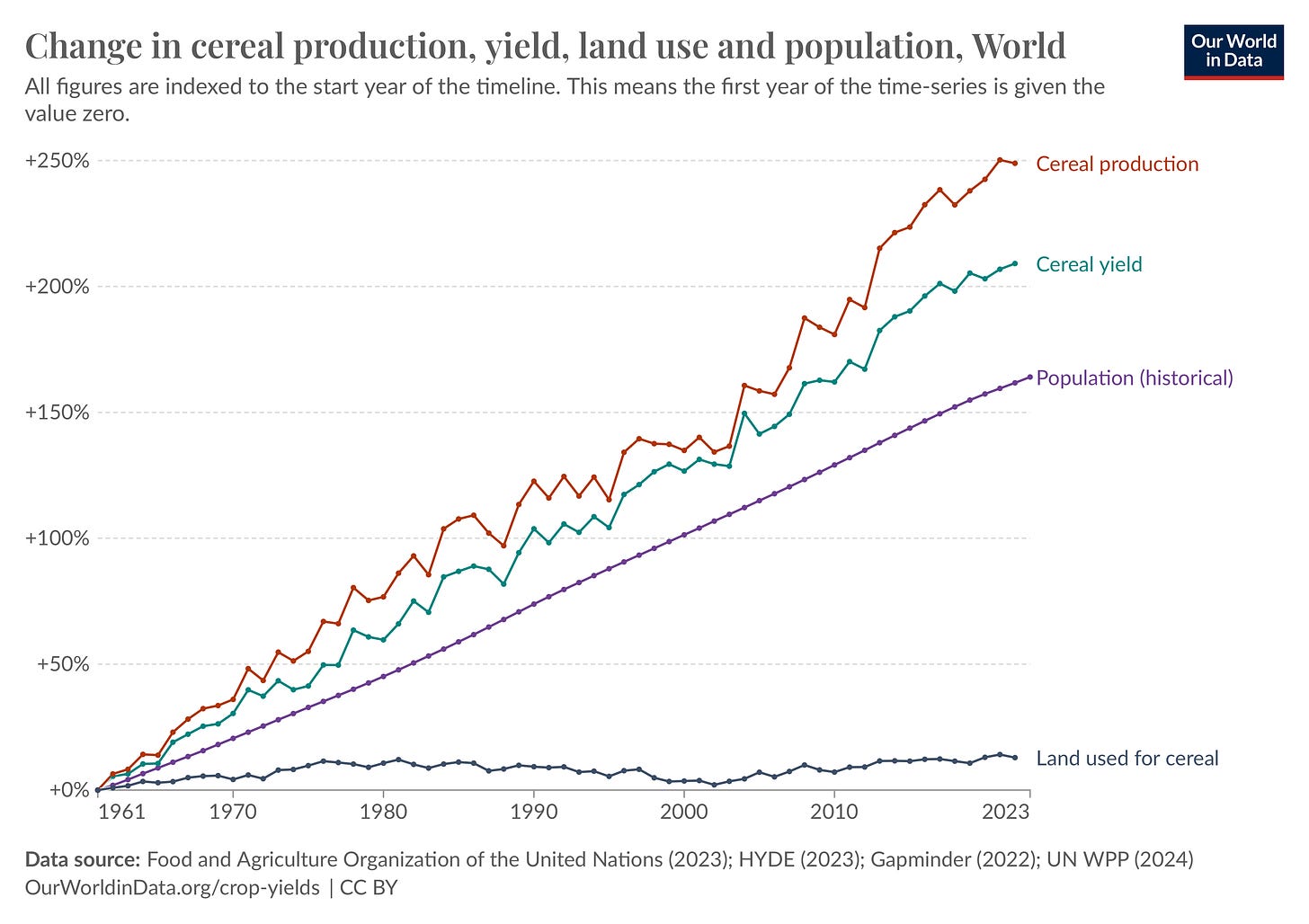
And finally, earlier this year, Gilead reported an antiretroviral drug, called lenacapavir, that was “100 percent protective” against HIV in a phase 3 trial of 5,300 women in South Africa and Uganda. This drug only needs to be given once every six months, meaning global health researchers can now — for the first time — begin to seriously think about eliminating HIV entirely.
These achievements are all the more remarkable because they happened in the veritable infancy of cell and molecular biology. Francis and Crick only worked out the structure of DNA in 1953, and the first genetically-modified organism was not patented until 1980. Even now, biologists are discovering entirely new classes of biomolecules; a few years ago, Ryan Flynn discovered glycoRNAs, which are sugar-tagged RNA molecules that seem to play important roles in cell signaling. Nobody had ever seen them before because methods to study RNA had been filtering them out.
The pace of biological progress is set to accelerate, too, because several organizations are already working on solving the “bottlenecks” I talked about earlier — making common biology experiments 100x cheaper, 10x faster, or much more replicable.
E11 Bio, for example, is a non-profit research group thinking seriously about how to cut costs associated with neuroscience research. They’re building a platform technology to map the entire mouse connectome, elucidating the connections between the neurons in this animal’s brain — all 100 million of them. Last year, the Wellcome Trust estimated it would take 17 years and cost $10 billion to do this project, but E11 hopes to develop technology that can do it for under $100 million; a 100x reduction in price.
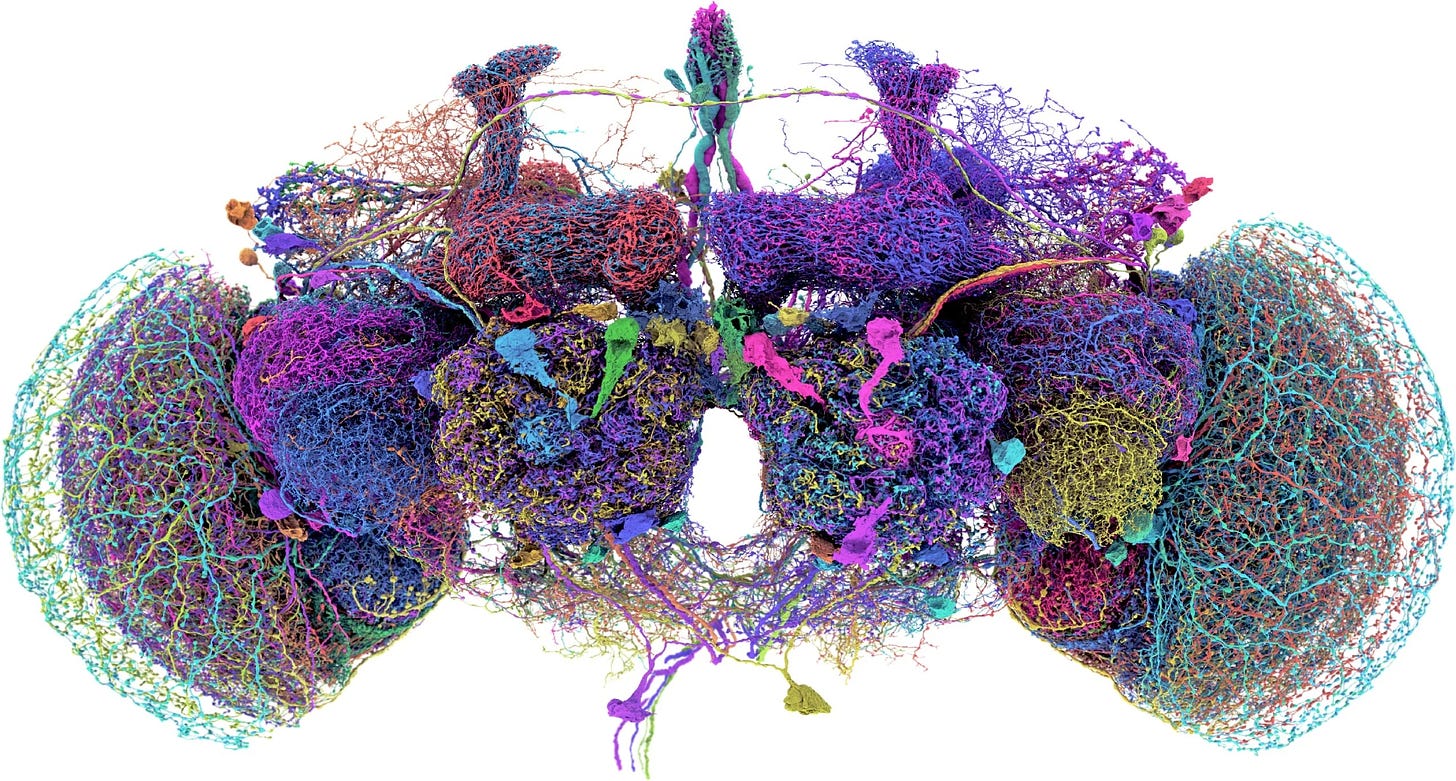
And Cultivarium, another non-profit, is collecting data specifically to train predictive models that can remove key bottlenecks associated with biology research on microbes (namely, most microbes don’t grow in the laboratory at all, and we often don’t know why). As I have previously written for Asimov Press:
As Cultivarium’s data swells, it also acts as a flywheel to drive future progress. The team now has enough data, for example, to train computational models that can predict optimal growth conditions for microbes, even when the microbe has never been cultured. The model ingests genome sequences and spits out predictions for a microbe’s desired oxygen, temperature, salinity (or saltiness) and pH levels …
…The model predicted oxygen tolerance with 92 percent accuracy and optimum temperatures with 73 percent accuracy… In general, the model was able to make accurate predictions even when just 10 percent of a microbe’s genome sequence was available. In other words, the model can make a reasonable assumption about growth conditions for entirely new taxonomic groups of microbes even when just a tiny part of its genome has been sequenced.
… As Cultivarium democratizes access to a broader palette of lifeforms, they hope that other researchers will continue their work, discover useful tools, and fuel the machines of progress.
While these developments accelerate specific types of biological experiments, true progress stems from applying our research findings to the real-world: vaccines, medicines, food, and more. In this essay, I’ve focused on bottlenecks that are limited to research at the bench, but solutions to those bottlenecks will not, on their own, be sufficient to make the world better, as Amodei also acknowledges.
I’m currently reading Gene Dreams, a book published in 1989 by Robert Teitelman. In the early days of biotechnology, writes Teitelman, speculators and investors tended to focus “on scientific wonders — on scientific possibilities — while skipping quickly across the more immediate, more contingent, technical, social, and business realities.”
Most of the early biotechnology companies failed; they over-promised and under-delivered, and not always because the science itself didn’t work. Teitelman continues:
A company that has mortgaged its future or lacks development skills or has no money left or has a product no one wants faces a limited future, no matter how powerful its technology. In the pursuit of truth, science needs no market; technology, on the other hand, has no reality beyond its application and exists only in relation to the marketplace.
Consider Sub1 rice, a genetically-enhanced variety of rice that carries a gene that enables the plants to survive prolonged flooding for up to two weeks. Normal rice typically dies after three days of flooding, meaning farmers in low-lying areas of southeastern Asia can (and do) lose their entire harvests during rainy years. Sub1 rice is now being planted in India, Bangladesh, and Nepal, but when these seeds were first distributed, many farmers weren’t able to access them because other farmers were unwilling to sell or trade with members of certain castes or communities. In short, culture is an oft-overlooked bottleneck.7
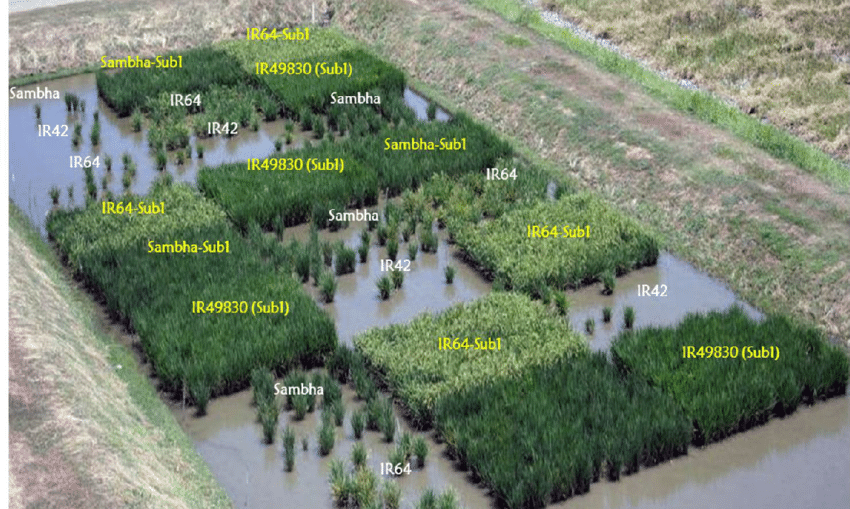
I don’t think that the bottlenecks discussed in this essay “need” AI to be solved. They can be addressed without Anthropic or DeepMind or OpenAI, but would likely be solved faster with them. But if we do manage to develop generally applicable methods to speed up biology experiments, or cheap and accessible measurement tools that collect far more data at once, then I suspect we’ll be able to train more capable AIs that can understand and design new types of biological systems.
Biology is awash in discovery and wonder, as Teitelman says, but actually bringing the fruits of that science into the world involves things beyond wonder. It involves pragmatism and cultural sensitivity. It demands protocols, regulations, and collaborative efforts between human beings. To that end, it isn't clear that technical hurdles themselves are the biggest challenge for biology this century; and that will hold true even with the emergence of a superintelligence.
Niko McCarty is founding editor of Asimov Press.
This essay is adapted from a talk I recently gave at the Roots of Progress conferencein Berkeley. If you have ideas for speeding up biological progress, please email niko@asimov.com. I’m considering turning these ideas into a full-length book and would appreciate your insights.
Thanks to Adam Marblestone, Sam Rodriques, Alec Nielsen, Dan Voicu, Merrick Pierson Smela and Xander Balwit for helpful comments.
Cite: Niko McCarty. “Levers for Biological Progress.” Asimov Press (2024). DOI: https://doi.org/10.62211/24kg-12rt
1 Marblestone writes: “My favorite example of this is we don't currently have connectomes for anything bigger than a fly so what can we really say about neural circuits.”
2 Cost is not inherently limiting for long-term progress. If future AIs are able to identify useful drugs, then the main costs of clinical trials — like patient recruitment — will be well worth spending the money on.
3 The human genome contains around 20,000 to 25,000 protein-coding genes, but exact numbers vary.
4 I visited the Institute for Protein Design earlier this year and asked them how much money they spend each month on DNA synthesis. They wouldn’t tell me exact numbers, but I suspect it’s in the millions.
5 Written in partnership with Julian Englert, CEO of AdaptyvBio.
6 Hi-C works by linking DNA together with formaldehyde and then sequencing the bits that remain stuck together.
7 Not to mention scaling things (i.e. growing drugs in 1,000-liter bioreactors), regulatory limits, and ethical quandaries.